Snowflake’s (NYSE:SNOW) Pros and Cons as the Modern Data Stack Evolves
Pros
-
To increase the speed at which data turns into business insights, enterprises need more data to be structured, and structured data is Snowflake’s core competence. Exploring raw, unstructured data is still valuable for data science use cases, but ultimately business decisions still need to be predicated on curated, structured data. Hence, OLAP workloads (i.e., queries against structured data in a data warehouse) will continue to hold the most value in the value chain. Therefore, as the lines blur between structured and unstructured, and between business intelligence and data science use cases, generally Snowflake should have the advantage over Databricks.
Snowflake’s (NYSE:SNOW) Pros and Cons as the Modern Data Stack EvolvesBrief History of the Journey to the Modern Data StackWhere Does Snowflake Operate in the MDS, and Where Is It Expanding ToSnowflake’s Competitive Position in the MDSGen AI: Snowflake vs DatabricksValuation ConsiderationsConclusion -
Unlike Databricks, SNOW owns the storage layer, which is empowering it with many benefits, such as:
-
-
optimizing the performance of OLAP workloads
-
providing higher levels of security and governance
-
facilitating a marketplace for data sharing
-
enabling vertical-specific data clouds
-
and developing an application building layer on top.
-
SNOW’s competitors that only own the compute layer are limited in how they can optimize performance and expand their TAM.
-
-
The promise of data applications, whereby applications are built atop data stores, should give SNOW the upper hand over Databricks. Applications of all types (consumer, B2B, enterprise AI) need to be built on curated data, which is SNOW’s core competence.
-
SNOW versus Databricks is a similar rivalry to Apple versus Google in the smartphone industry during the 2010s, as respectively, they are closed-source and open-source companies. During the 2010s, Apple delivered superior user experience by tightly integrating its software and hardware, and commanded a premium accordingly. Google played catch up by creating the open-source Android operating system to be used by smartphone makers, but because of the open nature, there was no standards for the app store nor the API service layer, resulting in poor user experience. In response, Google had to create more and more closed-source components to improve the quality, thus defeating the purpose of open-source. We are seeing Databricks do something similar to Google, which somewhat validates that SNOW’s approach is likely the best approach for the Modern Data Stack.
Cons
-
Contrary to the common investor perception, SNOW’s most dangerous competition is from that of startups in the open source community, rather than Redshift and BigQuery. For instance, instead of using SNOW, an enterprise could use a combo of internal data engineering resources, an open-source storage layer, and an open-core vendor’s compute layer, to construct something similar to SNOW. Done right, this would probably save costs (likely in the short-term but less likely over the long-term) but be unwieldy to manage. Therefore, it’s possible we see a bifurcation whereby we see different players emerge dominant in the low-end and high-end of the market. We may see many large enterprises with sufficient internal resources or enterprises with a short-to-medium term focus on cutting costs, will choose the open-source/open-core alternative stack. And those enterprise customers in the higher end of the market will choose SNOW for its superior end-to-end user experience.
-
SNOW’s original competitive advantage of storage and compute separation has long evaporated as it is now the industry standard. This means SNOW needs to take on some risky ventures to expand its ecosystem and raise the entry barriers further.
-
Compared to newer players, SNOW is weaker on the data source side of the Modern Data Stack, or MDS. As the demand for real-time data processing increases, as well as the demand for data mesh architectures that enable the querying of data at its source, SNOW could be at a disadvantage.
Brief History of the Journey to the Modern Data Stack
Since the computer age’s inception, data storage has continually evolved. In the mainframe era, magnetic tape was the primary storage mechanism, later replaced by disk storage. The 1970s saw the relational database (RDBMS) revolutionizing the industry, making it easier to map applications to databases, and propelling the IT boom.
Standards like SQL, the PC revolution, and the rise of the internet led to many new applications and systems, each requiring its own RDBMS. Subsequently, the mobile, cloud, and SaaS era brought further database fragmentation, resulting in data silos, hindering business analysis and leading to the downfall of some corporations.
The first data warehouse (DW) 1.0 provided a standardized, single source of truth for a company’s data, thus enabling efficient business analysis. Then, cloud-based DWs (CDW) or DW 2.0, initiated by AWS’ Redshift, eliminated upfront capital expenditure on servers.
SNOW introduced DW 3.0, revolutionizing the industry by separating storage and compute. An analogy could be a restaurant kitchen separating its cooking and storage spaces by utilizing outdoor/mobile cooking stations, thus efficiently increasing cooking capacity without expanding storage. However, what was originally SNOW’s advantage, is now industry standard.
Simultaneously, the rise of the Data Lake (DL) catered to the need for storing unstructured data from various sources like social media. AWS’ S3 and other hyperscalers’ object storage became known as DLs, offering cheap and scalable storage.
However, DLs lump various raw data altogether with little governance, and hence aren’t optimized for querying and retrieval. Moreover, DLs often turn into data swamps due to poor quality data. To unlock DL potential, structure and governance are required, to enable querying with languages like SQL.
This brings us to the current stage of the modern data stack evolution. All data has potential value, but it needs to be efficiently curated and standardized, making it ready for querying, analysis, and deriving business insights. In response to market demands, we are seeing a merger of DL and DW, enabling DW-like analysis on DL data. Databricks has expanded from big data and DL expertise towards DW, coining it Data Lakehouse. Similarly, SNOW expanded from its core CDW to DL, naming it Data Cloud.
Open-core players have entered the space, offering a storage layer for transforming raw data in the DL into useful datasets, and providing adaptable compute layers capable of analyzing various data sources and formats. The common goal is to empower enterprises to efficiently analyze all of their data, reduce costs, and increase data velocity for improved business outcomes.
Where Does Snowflake Operate in the MDS, and Where Is It Expanding To
To understand SNOW’s moat resilience we need to first clarify exactly where it operates in the fast evolving MDS (Modern Data Stack).
The DL and DW are the core of the MDS because they are the destinations for the majority of data. Data engineers build data pipelines to extract data from sources, process that data in either batches, micro batches, or in real-time, and then load the data into the DL or DW. Analysts, whether data scientists or business analysts, want to explore, search, and query the data in the DL/DW. To do so, they can execute the compute in large batch analysis, in an ad hoc manner, or by stream processing if they are analyzing real-time data.
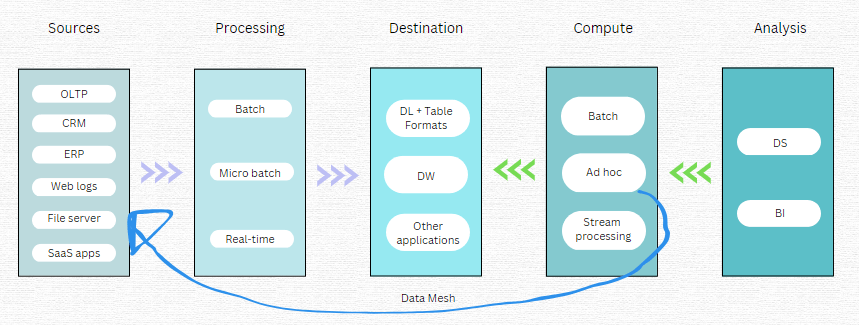
Convequity
SNOW operates on the right-hand side of the above diagram. Historically, they served the BI analyst needing to make batch or ad hoc query workloads, but mostly the latter, and those queries are executed against the CDW.
Historically, Databricks served data scientists, but across a broader scope. They provide a managed service for the open-source Spark, which is a big data framework for processing, compute, and analysis of data enroute to, or stored in, the DL. And whereas SNOW has focused on ad hoc, Databricks has catered for batch workloads. However, both data management vendors are now expanding from their core areas of expertise. They both aim to serve data scientists and BI analysts and provide each type of compute workload (batch, ad hoc, stream).
As previously mentioned, both have rearchitected their stack to facilitate their expansion plans. Databricks has created Delta Lake, which is an abstraction storage layer (labelled table format in the diagram) that sits atop the DL and transforms raw data into table formats, so that data can be efficiently analyzed. Combined, Databricks storage and compute layers form the Lakehouse. Snowflake has introduced native support for semi-structured and unstructured data that allows users to store raw data in the CDW and curate it later on ready for business analyst consumption. More recently, Snowflake has made significant progress in providing various native AI/ML tools for data scientists, so they can directly explore and analyze raw data inside of Snowflake.
Another development is that of combining OLTP (Online Transactional Processing) with OLAP (Online Analytical Processing). The former is a database that manages the insert, update, and delete operations of business-critical transactions, such as those related to finance. OLTPs are designed for simplicity and high performance because they need to process critical data in real-time. As depicted in the above diagram, they are usually a source to OLAP, which is a term commonly used for data in a DW. Traditionally, OLTP data is transformed from row to columnar formats and standardized ready for analytical OLAP workloads. However, Snowflake has brought both untransformed OLTP and OLAP together to deliver HTAP, or Hybrid Transactional Analytical Processing. This will speed up data velocity and reduce costs by putting less transformation strain on the data pipeline. If SNOW can be successful in storing native OLTP data for analytical purposes, it expands the TAM considerably from its core CDW because this is the bread and butter of Oracle’s multi-billion-dollar business.
An innovative development in which neither data giant has a foothold yet, is the data mesh, depicted in the above diagram. This is a new emerging way to make ad hoc queries, whereby the compute engine leverages APIs to make queries directly in the data source databases. Starburst, the commercial entity behind the open-source Trino, is a startup pioneering the data mesh architecture. The data mesh approach could save substantial costs for enterprises because they wouldn’t need to build as many data pipelines and do as many transformations of data. Additionally, the data mesh architecture enables analysts to interact with fresher data.
Snowflake’s Competitive Position in the MDS
Below we share our take on who are the key players competing against SNOW in the MDS. SNOW’s most like-for-like competitors are AWS’ Redshift and GCP’s BigQuery, as both own the storage and compute layer and are closed-source. For a long time, AWS’ Redshift was far inferior to SNOW, despite AWS owning the DL layer (S3). However, with the new architecture incorporating an acceleration layer based on AWS’ chips, Redshift is probably able to deliver a 1-2x performance gain versus SNOW on most workloads. But this probably isn’t enough to sway customers to switch from SNOW, and SNOW still has the unmatched end-to-end user experience with tons of out-the-box features. A similar assessment can be made for BigQuery versus SNOW. SNOW’s other key advantage is being multi-cloud, whereas Redshift only operates on AWS and BigQuery only operates on GCP.
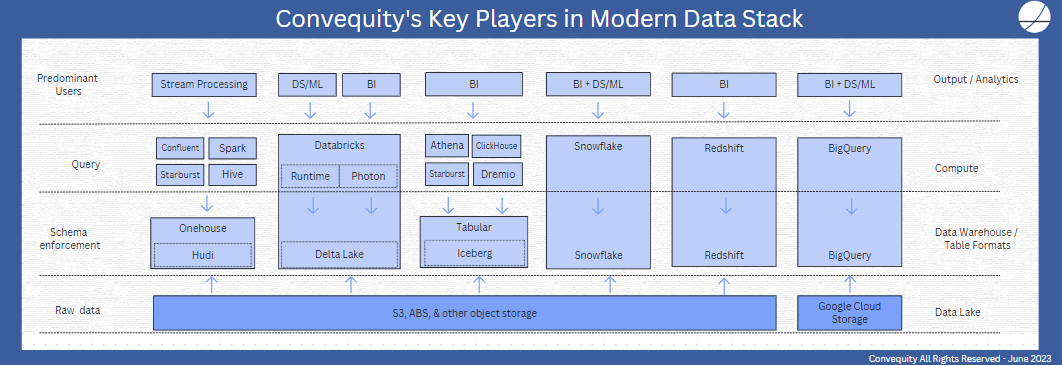
Convequity
SNOW’s toughest competition comes from Databricks with its compute layer Runtime (mainly for data science and machine learning) or Photon (designed for SQL-based business intelligence queries) and its table format storage layer Delta Lake, designed for DW-like analysis on DL (or an abstraction thereof). Databricks’ deep rooted problem, however, is that the company markets itself as the open-source alternative to SNOW, but then creates Delta Lake which lacks interoperability. Delta Lake is tightly integrated with Databricks’ Photon, and doesn’t work well with other compute engines. In essence, Databricks has Runtime (which is its Spark-as-a-Service, for those who have heard of open-source Apache Spark), Photon, and Delta Lake, which are built on open-source software but have many fundamental and valuable features in closed-source. Compared to SNOW, this is a fuzzy marketing message and inevitably introduces complexity and degrades user experience. By not being caught between open and closed source, like Google experienced during the 2010s, SNOW can deliver a more consistent and better quality user experience for all stakeholders.
For those larger enterprises with substantial data engineering resources and expertise, a combo of Starburst, Dremio, Clickhouse, or maybe AWS’ Athena, with Iceberg might be the preferred choice over SNOW. Iceberg is fast becoming the standard for open-source, table format storage, to enable SQL queries over DL. Unlike Delta Lake, which Databricks created to be predominantly operable with Photon, Iceberg has broad open-source community development and is completely independent from the compute layer. A couple of years ago, the founders of open-source Iceberg decided to found Tabular, which is a managed service for Iceberg.
Iceberg has spawned the likes of the aforementioned compute engines by lowering the entry barriers into the OLAP world. Starburst, Dremio, Clickhouse, and Athena are also driving the data mesh movement, whereby analysts can directly query the data at its source.
This creates more competition for SNOW at the compute layer, because, for instance, analysts can use Starburst to query data in Iceberg, which can be laid atop any object data store (aka DL), or use Starburst to query data at source, thus removing data pipeline ETL/ELT processing and saving huge costs. So these startups can potentially provide greater flexibility and cost savings versus SNOW. However, by owning the storage layer, SNOW still has plenty of opportunity to improve performance and expand into value adding adjacent markets, like data sharing and data apps. Furthermore, you can only guarantee a high level of security and governance, which are prerequisites for data sharing, if you own the storage layer.
SNOW has responded to an extent by enabling analysts to use SNOW to query data residing in Iceberg. This is potentially a smart move because while it supports the adoption of Iceberg, it slows down the momentum of the likes of Starburst, and slows down the momentum of Databricks’ Delta Lake and Photon. By allowing SNOW users to use Iceberg, it may lose some native storage but retain more compute, which is the higher value portion of the MDS.
Lastly, we’ll mention Hudi and Onehouse. Hudi is another open-source, table format, storage layer that sits on top of the DL, but is designed for processing data in real-time and making it queryable in real-time. Hudi was created internally at Uber as a way to add transactional operations to its flow of real-time data. In 2016, Hudi was open-sourced, and then in 2021 the same founders of Hudi created Onehouse as the commercial managed service of Hudi.
Hudi is tightly coupled with Spark, whereas Iceberg is more open and supportive of various tools. This means SNOW can’t provide external support for Hudi like it has for Iceberg, which may be a disadvantage because customers needing stream processing workloads on real-time data in Hudi will choose either Confluent (via its Jan-23 acquisition of Immerok, which is a managed service of Apache Flink), Spark, Hive, or Starburst.
Onehouse is the commercial entity backing Hudi but is operable with other table format storage layers, such as Delta and Iceberg. Onehouse’s objective is to be the connective tissue between the various DLs and open-source table formats, and empower all types of users to interact with data in the DL (whether in Hudi, Delta, or Iceberg) in a way that suits their use case. Currently, Hudi and Onehouse are more experimental, lack maturity, and have less open-source support than Iceberg, but as the need for real-time processing grows, they could become more of a competitor to SNOW’s ecosystem.
In summary, SNOW has more advantages than disadvantages amid this evolving MDS landscape. By being vertically integrated, SNOW can bring new products to market faster and provide an overall superior user experience. By owning the storage layer they can provide the necessary security and governance for data sharing among entities, and data rich applications and enterprise AI can be built on top. And where SNOW is at a disadvantage, like the open-source developments, it has responded by releasing support for external table formats.
Despite, these positives, however, as outlined in this report, SNOW’s moat is far less certain than what public investors generally assume, because Databricks, Starburst et al, can deliver lower cost alternatives that are on par in terms of performance. The bonus for SNOW investors, however, is that these alternatives have far greater overheads and may not deliver a lower TCO (Total Cost of Ownership) over the long-term.
Gen AI: Snowflake vs Databricks
Last month SNOW acquired Neeva, a search technology startup powered by LLMs (Large Language Models). Search is fundamental for how analysts interact with data, and the integration of Neeva will offer analysts a conversational paradigm to either replace or complement SQL-based workloads. LLM-based search could open another few billion dollars of TAM for SNOW. In essence, it has acquired a BoB keyword search capability comparable to Elastic’s ElasticSearch, but customers will be able to use it without the heavy overhead. Additionally, they will be able to fine tune their own LLMs using Neeva’s technology. Elastic’s $6bn market cap offers an inkling into how big the opportunity for SNOW could be.
Databricks is a pioneer in machine learning (ML) but not in the deep learning (DL) behind these LLMs. And as traditional ML is maturing but DL is still very early in its development, Databricks doesn’t have the edge that it had a few years ago. Databricks has open-sourced Dolly 2.0, which is its 12 billion parameter LLM. But really, they have just fine-tuned another company’s LLM with a new human-generated instruction/response dataset, crowdsourced by Databricks employees.
Literally, as I write this, news has just come out that Databricks has acquired MosaicML, a startup that enables customers to train LLMs on their own data, for $1.3bn. This is a huge premium compared to the last valuation of $136m in its last funding round in October 2021, and could be indicative as to how desperate Databricks is to establish themselves in the LLM space because they don’t really have the in-house deep learning expertise. MosaicML’s LLM model has 30 billion parameters and our initial takeaway is that its performance is probably mid-range among other top open-source models.
Overall this will make Databricks more competitive in AI and back up its previous (unsubstantiated) marketing hype. However, it is more of a DIY LLMops offering, while SNOW’s Neeva is focused on delivering an LLM-powered end product. Generally, we would say SNOW’s roadmap in regards to Gen AI is clearer.
Valuation Considerations
SNOW management’s long-term revenue guidance is $10bn by FY29 (year ending 31st January). FY23 revenue was $2.066bn, so to achieve the FY29 target SNOW would need to produce a CAGR of 30%. Given the economic outlook, SNOW might lower this guidance or remove it in the coming quarters.
Let’s go with a more conservative CAGR of 25%, which is reasonable when considering the LTM revenue growth was 60% and growth reacceleration after the macro headwinds are behind is possible, even likely. This will increase revenue to $7.9bn in FY29. Apply a 10x EV/S to the $7.9bn to arrive at $79bn of EV in FY29. Assuming SNOW’s FCF margin will be around 35% in FY29, this would equate to an EV/FCF of 28.5x. Then discount the $79bn back approximately six years at a 11% WACC to get present EV of $42bn. This is 20% lower than the EV at the time of writing of $53bn. Using the same parameters with $10bn of revenue in FY29, we calculate a present EV of SNOW’s EV today.
In summary, SNOW’s valuation is still high, but not ridiculously high anymore. If EV/S and EV/GP drop 30%+ then it would be attractive. The caveat, however, is that the lower guidance (just 34% for 2Q24) may lead to easy beats and share price surges in the coming quarters.
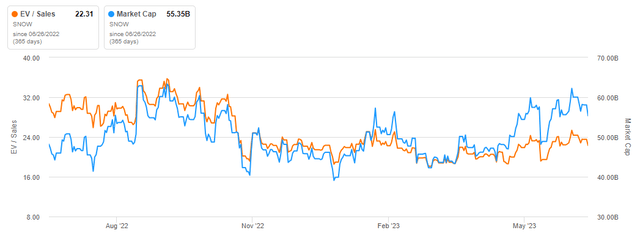
Seeking Alpha
Conclusion
SNOW’s moat isn’t as protected as the general investor community believes, because beside Redshift and BigQuery, there is mounting competition for SNOW, from Databricks and innovative startups. However, no alternative stack can produce the 5-10x performance gains needed to disrupt SNOW and compel customers to switch. Furthermore, its closed-source advantages are currently outweighing its disadvantages of not being in the open-source community. And despite its CDW background, SNOW seems to have a stronger footing in the Gen AI than its big data rival Databricks. These advantages position it well to benefit from the continued MDS evolution.
In regards to the valuation, SNOW stock is overpriced but the long-term thesis is still intact; we’re waiting for the stock to drop 20-30% before gradually building a position.
Read the full article here